Keynotes
Keynote Speaker
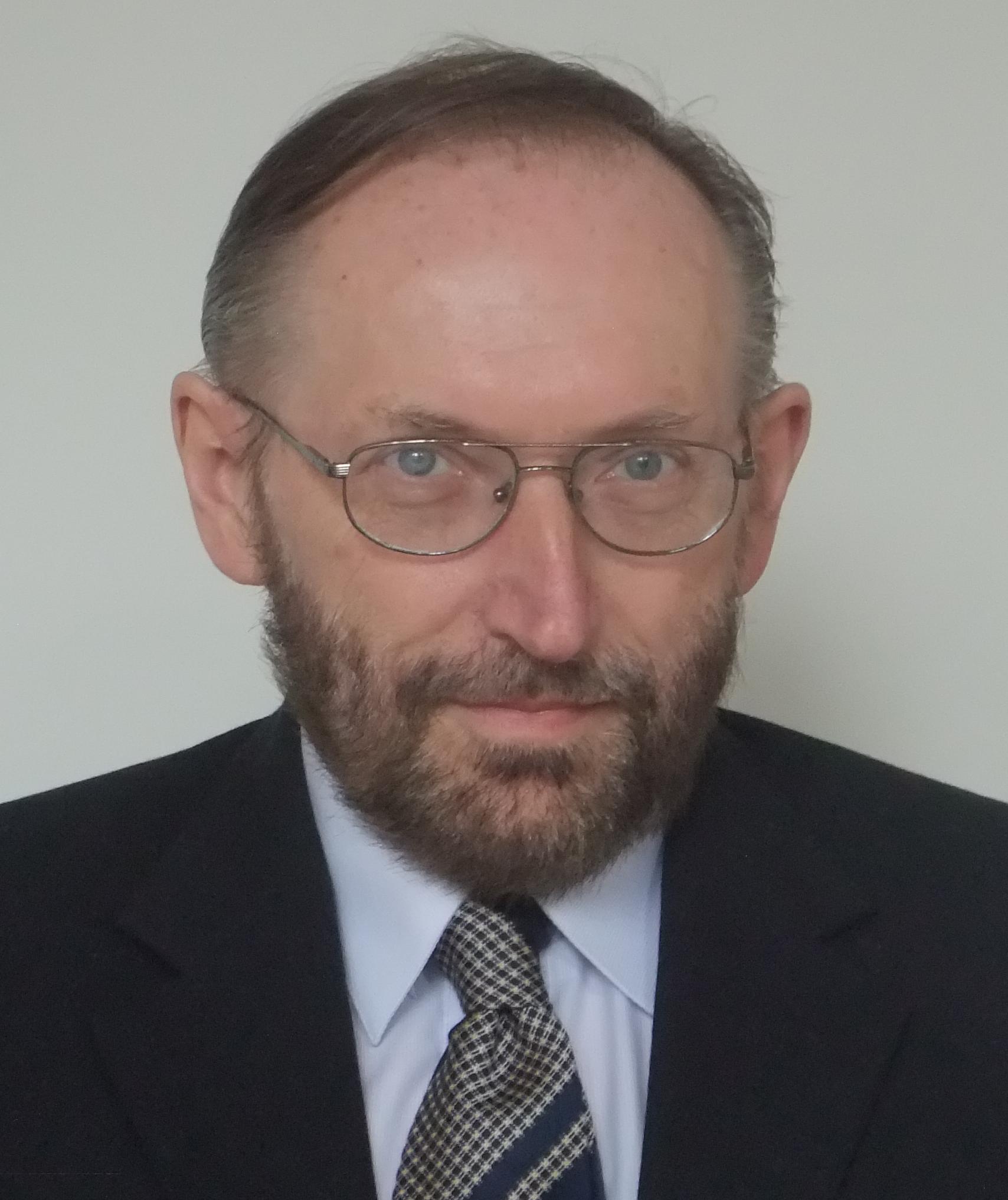
Prof. Witold Pedrycz
Department of Electrical & Computer Engineering
University of Alberta, Edmonton, Canada
Title: Credibility of Machine Learning Through Information Granularity
Abstract: Over the recent years, we have been witnessing numerous and far-reaching developments and applications of Machine Learning (ML).
Efficient and systematic design of their architectures is important. Equally important are comprehensive evaluation mechanisms aimed at
the assessment of the quality of the obtained results. The credibility of ML models is also of concern to any application, especially the one
exhibiting a high level of criticality commonly encountered in autonomous systems and critical processes of decision-making. With this regard,
there are a number of burning questions: how to quantify the quality of a result produced by the ML model? What is its credibility?
How to equip the models with some self-awareness mechanism so careful guidance for additional supportive
experimental evidence could be triggered?
Proceeding with a conceptual and algorithmic pursuits, we advocate that these problems could be formalized in the settings of Granular Computing (GrC).
We show that any numeric result be augmented by the associated information granules being viewed as an essential vehicle to quantify credibility.
A number of key formalisms explored in GrC are explored, namely those involving probabilistic, interval, and fuzzy information granules.
Depending on the formal settings, confidence levels and confidence intervals or coverage and specificity criteria are discussed in depth and we show
their role as descriptors of credibility measures.
The general proposals of granular embedding and granular Gaussian Process models are discussed along with their ensemble architectures.
In the sequel, several representative and direct applications arising in the realm of transfer learning,
knowledge distillation, and federated learning are discussed.
Bio: Witold Pedrycz (IEEE Life Fellow) is Professor in the Department of Electrical and Computer Engineering,
University of Alberta, Edmonton, Canada. He is also with the Systems Research Institute of the Polish Academy of Sciences,
Warsaw, Poland. Dr. Pedrycz is a foreign member of the Polish Academy of Sciences and a Fellow of the Royal Society of Canada.
He is a recipient of several awards including Norbert Wiener award from the IEEE Systems, Man, and Cybernetics Society,
IEEE Canada Computer Engineering Medal, a Cajastur Prize for Soft Computing from the European Centre for Soft Computing, a Killam Prize,
a Fuzzy Pioneer Award from the IEEE Computational Intelligence Society, and 2019 Meritorious Service Award from the IEEE Systems Man and
Cybernetics Society.
His main research directions involve Computational Intelligence, Granular Computing, and Machine Learning, among others.
Professor Pedrycz serves as an Editor-in-Chief of Information Sciences, Editor-in-Chief of WIREs Data Mining and Knowledge Discovery (Wiley),
and Co-editor-in-Chief of Int. J. of Granular Computing (Springer) and J. of Data Information and Management (Springer).
Keynote Speaker
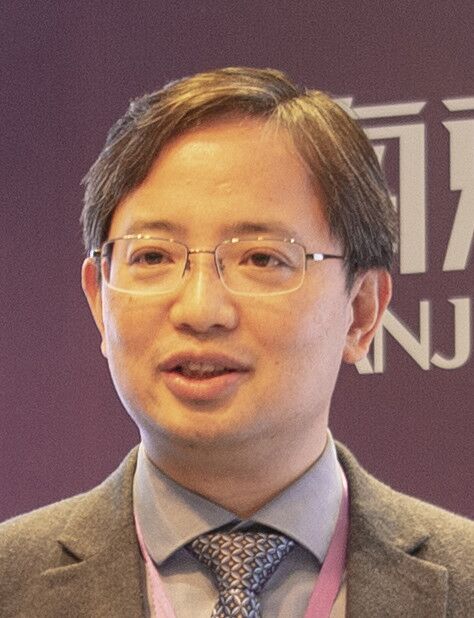
Prof. Zhi-Hua Zhou
Department of Computer Science & Technology, School of Artificial Intelligence
Nanjing University, China
Title: A new paradigm to leverage formalized knowledge and machine learning
Abstract: To develop a unified framework which accommodates and enables machine learning and logical knowledge reasoning to work together effectively is a well-known holy grail problem in artificial intelligence. It is often claimed that advanced intelligent technologies can emerge when machine learning and logical knowledge reasoning can be seamlessly integrated as human beings generally perform problem-solving based on the leverage of perception and reasoning, where perception corresponds to a data-driven process that can be realized by machine learning whereas reasoning corresponds to a knowledge-driven process that can be realized by formalized reasoning. This talk ill present a recent study in this line.
Bio: Zhi-Hua Zhou is Professor of Computer Science and Artificial Intelligence at Nanjing University. His research interests are mainly in machine learning and data mining, with significant contributions to ensemble learning, multi-label and weakly supervised learning, etc. He has authored the books "Ensemble Methods: Foundations and Algorithms", "Machine Learning", etc., and published more than 200 papers in top-tier journals or conferences. Many of his inventions have been successfully transferred to industry. He founded ACML (Asian Conference on Machine Learning), served as Program Chair for AAAI-19, IJCAI-21, etc., General Chair for ICDM'16, SDM'22, etc., and Senior Area Chair for NeurIPS and ICML. He is series editor of Springer LNAI, on the advisory board of AI Magazine, and serves as editor-in-chief of Frontiers of Computer Science, associate editor of AIJ, MLJ, IEEE TPAMI, ACM TKDD, etc. He is a Fellow of the ACM, AAAI, AAAS, IEEE, and recipient of the National Natural Science Award of China, the IEEE Computer Society Edward J. McCluskey Technical Achievement Award, the CCF-ACM Artificial Intelligence Award, etc.
Keynote Speaker
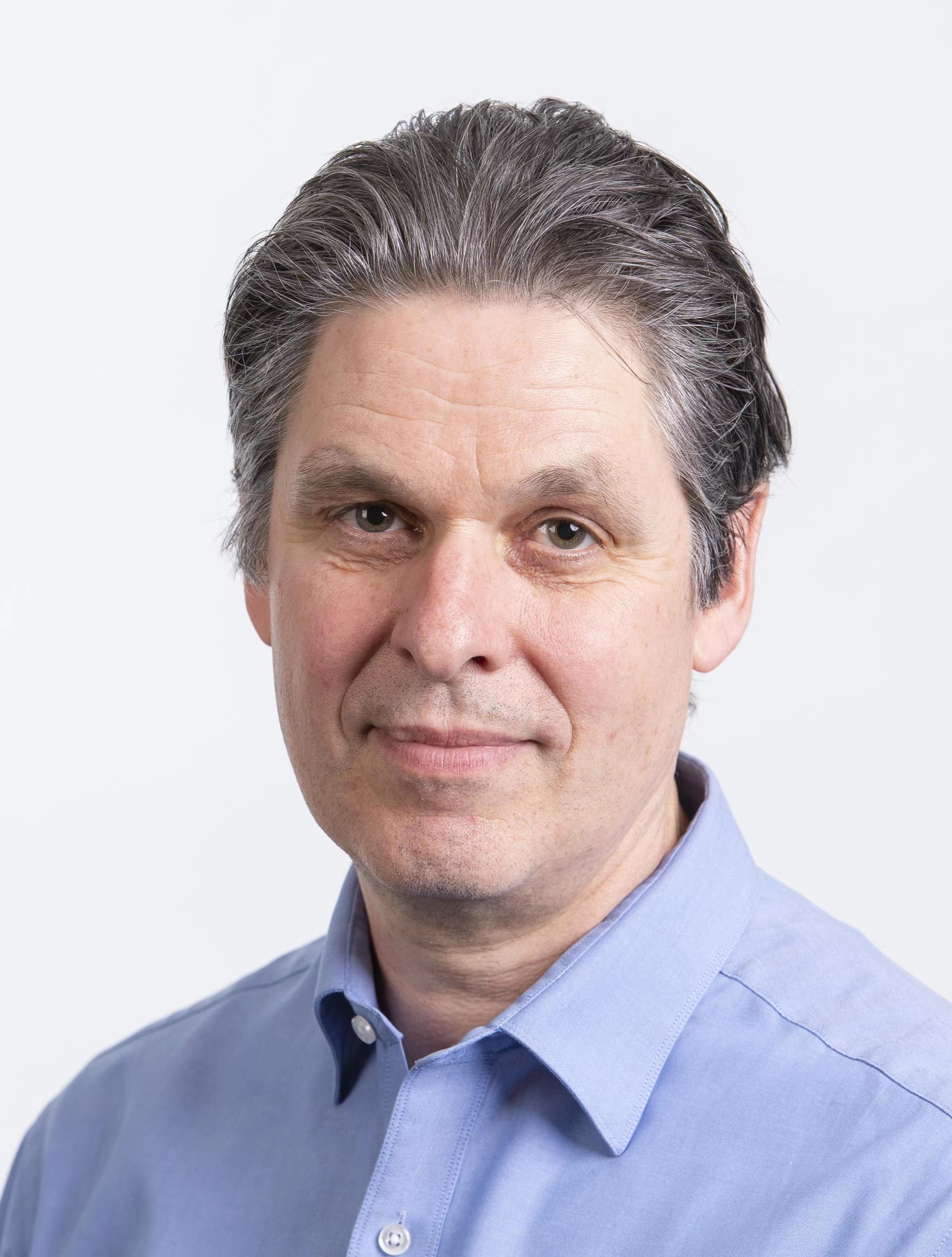
Prof. Geoff Webb
Department of Data Science & Artificial Intelligence, Monash Data Futures Institute
Monash University, Australia
Title: Recent Advances in Assessing Time Series Similarity Through Dynamic Time Warping
Abstract: Time series are a ubiquitous data type that capture information as it evolves over time. Dynamic Time Warping is the classic technique for quantifying similarity between time series. This talk outlines our impactful program of research that has transformed the state of the art in practical application of Dynamic Time Warping to big data tasks. These include fast and effective lower bounds, fast dynamic programming methods for calculating Dynamic Time Warping, and intuitive and effective variants of Dynamic Time Warping that moderate its sometimes-excessive flexibility.
Bio: Professor Geoff Webb is an eminent and highly-cited data scientist. He was editor in chief of the Data Mining and Knowledge Discovery journal, from 2005 to 2014. He has been Program Committee Chair of both ACM SIGKDD and IEEE ICDM, as well as General Chair of ICDM and member of the ACM SIGKDD Executive. He is a Technical Advisor to machine learning as a service startup BigML Inc and to recommender systems startup FROOMLE. He developed many of the key mechanisms of support-confidence association discovery in the 1980s. His OPUS search algorithm remains the state-of-the-art in rule search. He pioneered multiple research areas as diverse as black-box user modelling, interactive data analytics and statistically-sound pattern discovery. He has developed many useful machine learning algorithms that are widely deployed. His many awards include IEEE Fellow, the inaugural Eureka Prize for Excellence in Data Science (2017) and the Pacific-Asia Conference on Knowledge Discovery and Data Mining Distinguished Research Contributions Award (2022).
Keynote Speaker
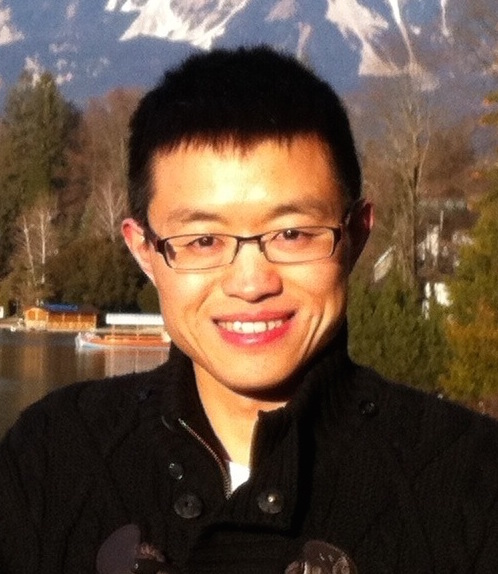
Prof. Jie Tang
Department of Computer Science
Tsinghua University, China
Title: ChatGLM: Run your own “ChatGPT” on a laptop
Abstract:
Large language models have substantially advanced the state of the art in various AI tasks, such as natural language understanding and text generation, and image processing, multimodal modeling. In this talk, I am going to talk about how we build GLM-130B, a bilingual (English and Chinese) pre-trained language model with 130 billion parameters. It is an attempt to open-source a 100B-scale model at least as good as GPT-3 and unveil how models of such a scale can be successfully pre-trained. Based on GLM-130B, we have developed ChatGLM, an alternative to ChatGPT. A small version, ChatGLM-6B, is opened with weights and codes. It can be deployed with one RTX 2080 Ti (11G) GPU, which makes it possible for everyone to deploy a ChatGPT! It has attracted over 2,000,000 downloads on Hugging Face in one month, and won the trending #1 model for two weeks.
GLM-130B: https://github.com/THUDM/GLM-130B
ChatGLM: https://github.com/THUDM/ChatGLM-6B
Bio: Jie Tang is a WeBank Chair Professor of the Department of Computer Science at Tsinghua University. He is a Fellow of the ACM, a Fellow of AAAI, and a Fellow of IEEE. His interests include artificial general intelligence, data mining, social networks, and machine learning. He served as General Co-Chair of WWW’23, and PC Co-Chair of WWW’21, CIKM’16, WSDM’15, and EiC of IEEE T. on Big Data and AI Open J. He is leading several major efforts on building Large Language Models, e.g., GLM-130B, ChatGLM, CogView/CogVideo, CodeGeeX. He also invented AMiner.cn, which has attracted 30 million users from 220 countries/regions in the world. He was honored with the SIGKDD Test-of-Time Award, the 2nd National Award for Science&Technology, NSFC for Distinguished Young Scholar, and SIGKDD Service Award.
Keynote Speaker
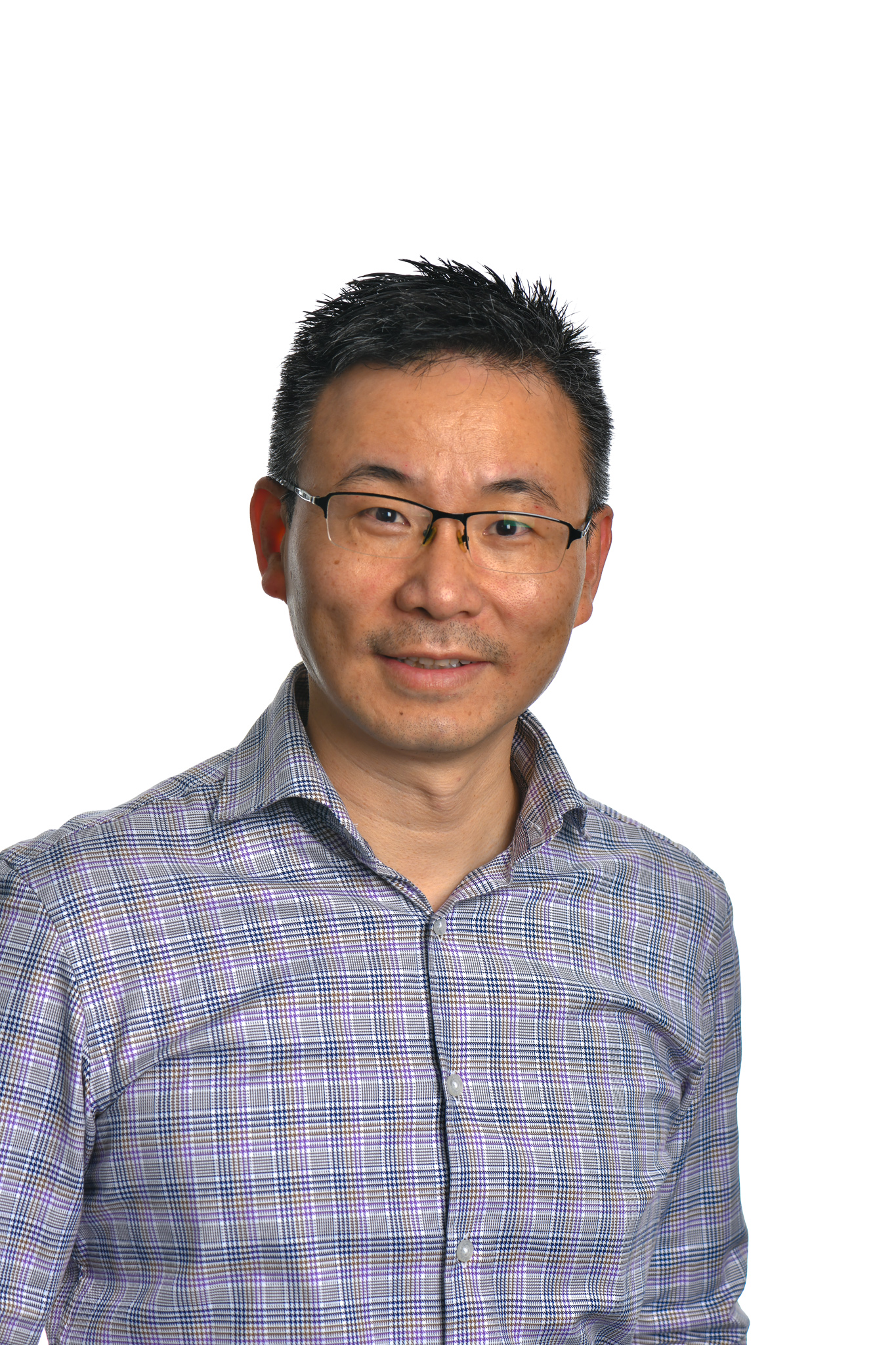
Prof. Gang Li
Strategic Research Center for Cyber Resilience and Trust (CREST)
Deakin University, Australia
Title: Topological Data Analysis : Discriminative Representations for Persistence Diagram
Abstract:
Topological data analysis (TDA) extracts topological features to quantify the shape of data. TDA has found applications in many fields, including computer vision. In TDA, persistence diagrams (PDs) are topological descriptors of data. However, PDs are not vector so they cannot be directly used in machine learning methods.
Recent efforts have transformed PDs into vectors to enable machine learning tasks (classification, regression, dimension reduction, etc). However, existing methods highly depend on pre-defined polynomials to map PDs to vector representations. This presentation introduces two recent advances in PD representation: polynomial representations and Hilbert space embeddings. These representations could extract more discriminative topological features without requiring pre-defined polynomials.
The representations enable applying various machine learning tasks to PDs and extracting insights from different types of topological datasets. Our work seeks to open new research directions and applications of TDA in chemical/biological science, and materials engineering.
Bio:
Prof. Gang Li is the AI director in the Strategic Research Center for Cyber Resilience and Trust (CREST) at Deakin University, Australia. His research includes data privacy, robust machine learning, group behavior analysis, and business intelligence. According to Google Scholar, his h-index is 37 with 6700+ citations with 12 papers receiving 100+ citations in Google Scholar. He holds one international patent, and his research has been funded by various agencies, including ARC, India DST Sparc Scheme and Hong Kong GRF.
Prof. Li serves as the chair for IEEE Task Force on Educational Data Mining (2020-2023), and on the IEEE Data Mining and Big Data Analytics Technical Committee (2017-2019 Vice Chair), IEEE Enterprise Information Systems Technical Committee.